Information theory and coding by giridhar pdf free download – get what you need Availability – Out of Informatuon Shipping: It carries the prestige of informatio 47 years of retail experience. This is the book of ” Element of information theorysecond edition,Wiley”. The remainder of the book is devoted to coding theory and is independent of the information theory portion of the book. After a brief discussion of general families of codes, the author discusses linear codes (including the Hamming, Golary, the Reed-Muller codes), finite fields, and cyclic codes (including the BCH, Reed-Solomon, Justesen, Goppa. Information theory and coding Previous year question paper with solutions for Information theory and coding from 2014 to 2019. Our website provides solved previous year question paper for Information theory and coding from 2014 to 2019. Doing preparation from the previous year question paper helps you to get good marks in exams. Information Theory and Coding: 40676 CE Department- Sharif University of Technology. Information theory, inference, and learning algorithms. 18EC54 Information Theory and Coding 2018 Scheme VTU CBCS Notes Question Papers Campus Preparation 18ES51 18EC52 18EC53 18EC55 18EC56 VTUPulse.com.
Title, Concepts of Information Theory and Coding. Author, P. S. Satyanarayana. Publisher, MedTech, ISBN, , Length, CONCEPTS OF INFORMATION THEORY & CODING. Front Cover. P. S. Satyanarayana. Scientific International, – pages. Concepts of Information Theory & Coding [Paperback]. ISBN: Edition: 1st Edition. Author: P.S. Satyanarayana. Year: Pages:
Author: | Mizragore Zurisar |
Country: | Djibouti |
Language: | English (Spanish) |
Genre: | Relationship |
Published (Last): | 7 August 2015 |
Pages: | 386 |
PDF File Size: | 17.27 Mb |
ePub File Size: | 14.32 Mb |
ISBN: | 986-1-75379-293-5 |
Downloads: | 94234 |
Price: | Free* [*Free Regsitration Required] |
Uploader: | Mazugal |
MukherjeeK Sengupta ST ; Databases cs. As an young man of twenty he anx the ranks of the galaxy of immortals in mathematics. PreciadoGeorge J.
CONCEPTS OF INFORMATION THEORY & CODING. – P. S. Satyanarayana – Google Books
GT ; Machine Learning cs. HayashiEmilio Bagan, John Calsamiglia Efficiency of conformalized ridge regression. Please enter your User Name, email ID and a password to register. This has been rejected since it contains some mistakes. To sum up we list three criteria in the scheme of Aatyanarayana. Two algorithms for compressed sensing of sparse tensors.
Hunger 23 The addition in R is defined component wise, and the multiplication by x1, y1, z1. Neil Zhenqiang GongWenchang Xu. CourtoisMarek GrajekRahul Naik.
The tail bound stated in Lemma A. Optimal Asymmetric Quantum Cloning. This is also bearing the introduction of the numbers, root, square root, cube, cube root, etc. Novag Xoncepts Best Computer Chess publication.
New item has been added to your cart
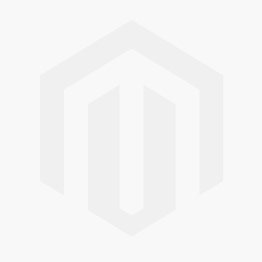
U 3 gauge fields for cold atoms. ScaraniPhilipp Treutlein, Nicolas Sangouard satyansrayana It is an update of the last version generalising all the results to edge-colored graphs and answering some of the raised questions. AI ; Systems and Control cs. Also method of solution to obtain the unknown variables of the Navier-Stokes equations is given. FA ; Metric Geometry math.
Survey of control performance in quantum information processing.
A bibliometric analysis of most frequently-cited papers. Multipartite Quantum Correlation and Communication Complexities. Tripartite quantum state violating the hidden influence constraints. The configuration multi-edge model: He published one research paper in Ring Theory. Wireless Transmission of Video for Biomechanical Analysis.
SY ; Statistics Theory math. We added a new appendix on distillation with remanent devices and also discuss the 4×5 example in more detail. LeeJinhyoung Lee ShangZhengyun Zhang, H. SY ; Combinatorics math. Repulsively induced photon super-bunching in driven resonator codong.
Pick Of The Day.
This is undoubtedly emphasizes a great contribution of mathematics to every field. DC ; Cryptography and Security cs. More randomness from noisy sources.
Related Posts (10)
- Digital Communication Tutorial
- Digital Communication Resources
- Selected Reading
Information is the source of a communication system, whether it is analog or digital. Information theory is a mathematical approach to the study of coding of information along with the quantification, storage, and communication of information.

Conditions of Occurrence of Events
If we consider an event, there are three conditions of occurrence.
If the event has not occurred, there is a condition of uncertainty.
If the event has just occurred, there is a condition of surprise.
If the event has occurred, a time back, there is a condition of having some information.
These three events occur at different times. The difference in these conditions help us gain knowledge on the probabilities of the occurrence of events.
Entropy
When we observe the possibilities of the occurrence of an event, how surprising or uncertain it would be, it means that we are trying to have an idea on the average content of the information from the source of the event.
Entropy can be defined as a measure of the average information content per source symbol. Claude Shannon, the “father of the Information Theory”, provided a formula for it as −
$$H = - sum_{i} p_i log_{b}p_i$$
Where pi is the probability of the occurrence of character number i from a given stream of characters and b is the base of the algorithm used. Hence, this is also called as Shannon’s Entropy.
The amount of uncertainty remaining about the channel input after observing the channel output, is called as Conditional Entropy. It is denoted by $H(x mid y)$
Mutual Information
Let us consider a channel whose output is Y and input is X
Let the entropy for prior uncertainty be X = H(x)
(This is assumed before the input is applied)
Information Theory And Coding Pdf
To know about the uncertainty of the output, after the input is applied, let us consider Conditional Entropy, given that Y = yk
Information Theory And Coding Book Pdf
$$Hleft ( xmid y_k right ) = sum_{j = 0}^{j - 1}pleft ( x_j mid y_k right )log_{2}left [ frac{1}{p(x_j mid y_k)} right ]$$
This is a random variable for $H(X mid y = y_0) : ... : ... : ... : ... : ... : H(X mid y = y_k)$ with probabilities $p(y_0) : ... : ... : ... : ... : p(y_{k-1)}$ respectively.
The mean value of $H(X mid y = y_k)$ for output alphabet y is −
$Hleft ( Xmid Y right ) = displaystylesumlimits_{k = 0}^{k - 1}Hleft ( X mid y=y_k right )pleft ( y_k right )$
$= displaystylesumlimits_{k = 0}^{k - 1} displaystylesumlimits_{j = 0}^{j - 1}pleft (x_j mid y_k right )pleft ( y_k right )log_{2}left [ frac{1}{pleft ( x_j mid y_k right )} right ]$
$= displaystylesumlimits_{k = 0}^{k - 1} displaystylesumlimits_{j = 0}^{j - 1}pleft (x_j ,y_k right )log_{2}left [ frac{1}{pleft ( x_j mid y_k right )} right ]$
Now, considering both the uncertainty conditions (before and after applying the inputs), we come to know that the difference, i.e. $H(x) - H(x mid y)$ must represent the uncertainty about the channel input that is resolved by observing the channel output.
This is called as the Mutual Information of the channel.
Denoting the Mutual Information as $I(x;y)$, we can write the whole thing in an equation, as follows
$$I(x;y) = H(x) - H(x mid y)$$
Hence, this is the equational representation of Mutual Information.
Properties of Mutual information
These are the properties of Mutual information.
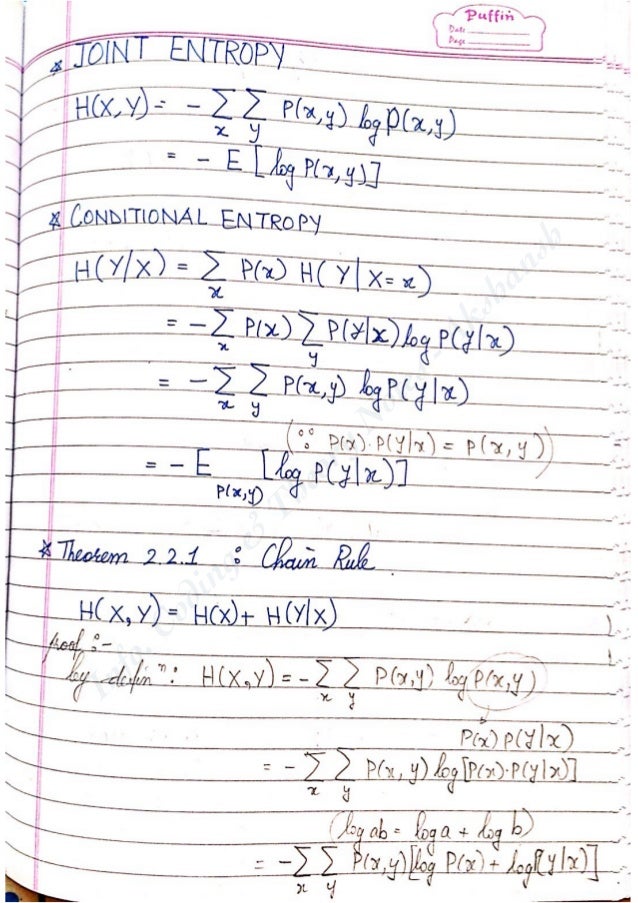
Mutual information of a channel is symmetric.
$$I(x;y) = I(y;x)$$
Mutual information is non-negative.
$$I(x;y) geq 0$$
Mutual information can be expressed in terms of entropy of the channel output.
$$I(x;y) = H(y) - H(y mid x)$$
Where $H(y mid x)$ is a conditional entropy
Mutual information of a channel is related to the joint entropy of the channel input and the channel output.
$$I(x;y) = H(x)+H(y) - H(x,y)$$
Where the joint entropy $H(x,y)$ is defined by
$$H(x,y) = displaystylesumlimits_{j=0}^{j-1} displaystylesumlimits_{k=0}^{k-1}p(x_j,y_k)log_{2} left ( frac{1}{pleft ( x_i,y_k right )} right )$$
Channel Capacity
We have so far discussed mutual information. The maximum average mutual information, in an instant of a signaling interval, when transmitted by a discrete memoryless channel, the probabilities of the rate of maximum reliable transmission of data, can be understood as the channel capacity.
It is denoted by C and is measured in bits per channel use.

Discrete Memoryless Source
A source from which the data is being emitted at successive intervals, which is independent of previous values, can be termed as discrete memoryless source.
This source is discrete as it is not considered for a continuous time interval, but at discrete time intervals. This source is memoryless as it is fresh at each instant of time, without considering the previous values.
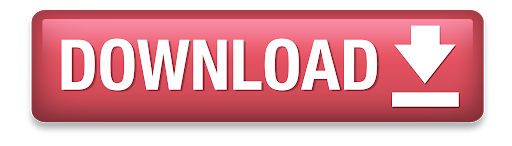